How To Find The Mean Absolute Deviation

The mean absolute deviation (MAD) is a statistical measure that calculates the average distance between each data point and the mean of the dataset. It is a useful tool for understanding the spread or dispersion of a dataset. To find the mean absolute deviation, you need to follow a series of steps that involve calculating the mean of the dataset, finding the absolute deviation of each data point from the mean, and then averaging these deviations.
Understanding the Concept of Mean Absolute Deviation

The mean absolute deviation is an important concept in statistics because it provides a measure of the variability of a dataset. Unlike the standard deviation, which can be influenced by extreme values (outliers) due to its use of squared differences, the MAD uses absolute differences, making it more robust to outliers. This characteristic makes the MAD particularly useful for datasets that contain outliers or for situations where a more robust measure of dispersion is needed.
Step-by-Step Process to Calculate the Mean Absolute Deviation
To calculate the mean absolute deviation, follow these steps:
- Step 1: Calculate the Mean of the Dataset - First, you need to find the mean (average) of your dataset. This is done by summing up all the values in the dataset and then dividing by the number of values. The formula for the mean is: mean = (sum of all values) / (number of values).
- Step 2: Calculate the Absolute Deviation of Each Data Point - For each data point in the dataset, subtract the mean and take the absolute value of the result. This gives you the absolute deviation of each point from the mean. The formula for the absolute deviation of a data point is: |data point - mean|.
- Step 3: Calculate the Mean Absolute Deviation - Finally, to find the mean absolute deviation, you average the absolute deviations calculated in the previous step. This is done by summing up all the absolute deviations and then dividing by the number of data points. The formula for the mean absolute deviation is: MAD = (sum of absolute deviations) / (number of data points).
Dataset Values | Absolute Deviations |
---|---|
Value 1 | |Value 1 - Mean| |
Value 2 | |Value 2 - Mean| |
... | ... |
Value N | |Value N - Mean| |
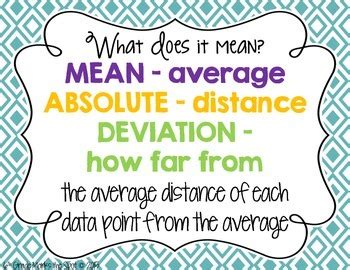
Interpretation and Application of Mean Absolute Deviation

The mean absolute deviation is a versatile statistic that can be used in various applications, including data analysis, finance, and quality control. A smaller MAD indicates that the data points are closer to the mean, suggesting less variability, while a larger MAD indicates greater variability. This measure can be particularly useful when the dataset contains outliers, as it provides a more robust measure of dispersion compared to the standard deviation.
Key Points
- The mean absolute deviation is a measure of the average distance between each data point and the mean of the dataset.
- It is calculated by finding the mean, then calculating the absolute deviation of each data point from the mean, and finally averaging these deviations.
- The MAD is a robust measure of dispersion, particularly useful when dealing with datasets that contain outliers.
- It can be used in various fields, including data analysis, finance, and quality control, to understand the variability of a dataset.
- A smaller MAD value indicates less variability, while a larger MAD value indicates greater variability in the dataset.
Common Applications of Mean Absolute Deviation
The mean absolute deviation has a range of applications due to its ability to provide a robust measure of data dispersion. It is commonly used in financial analysis to measure the volatility of investment returns, in quality control to monitor the consistency of manufacturing processes, and in data analysis to understand the spread of data points around the mean.
In conclusion, the mean absolute deviation is a valuable statistical tool that offers insights into the dispersion of a dataset. Its calculation involves straightforward steps, and its interpretation can provide meaningful insights into the variability of data. Whether in academic research, financial analysis, or quality control, understanding and applying the mean absolute deviation can enhance the understanding of data and support informed decision-making.
What is the primary advantage of using the mean absolute deviation over the standard deviation?
+The primary advantage of using the mean absolute deviation (MAD) is its robustness to outliers. Unlike the standard deviation, which uses squared differences and can be heavily influenced by extreme values, the MAD uses absolute differences, making it a more reliable measure of dispersion in datasets containing outliers.
How does the mean absolute deviation relate to the concept of data variability?
+The mean absolute deviation is a direct measure of data variability. It quantifies the average distance of data points from the mean, providing insight into how spread out the data is. A higher MAD value indicates greater variability, while a lower MAD value suggests less variability.
What are some common applications of the mean absolute deviation in real-world scenarios?
+The mean absolute deviation has various applications, including financial analysis to measure portfolio risk, quality control to monitor process variability, and data analysis to understand the spread of data points. Its robustness to outliers makes it particularly useful in scenarios where data may contain extreme values.